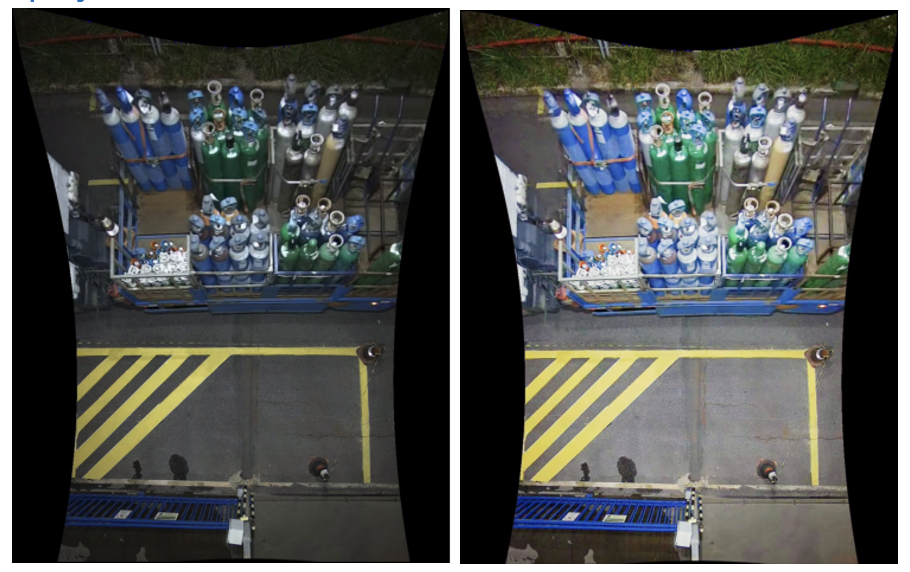
The Air Liquide use case – Cylinder counting
A use case for automatic object counting in adverse weather conditions
Functional sets /
Issues addressed:
Context
To learn, machine learning models need to train on an immense amount of data. Ideally, their learning should cover all possible scenarios. But unpredictable scenarios inexorably appear in an industrial context where human and environmental factors intervene. In addition, some data is difficult to obtain and use. The challenge therefore lies in the ability to propose machine learning models that maintain their operation in scenarios for which no or little real data is available. Synthetic data, produced artificially by generative AI approaches, thus represents an effective response to this problem and a means, for industrial players, to create more efficient and more robust AI models.
Detailed description of the use case and the problem to be resolved
Air Liquide uses cameras equipped with an AI-based system for automatic outdoor inventory counting. Used during the day and in sunny weather, this counting tool is significantly less efficient at night or in bad weather (e.g. rain, snow). The challenge to be taken up is therefore multiple: how to detect these new scenarios in real time, carry out a sensitivity analysis of the system in the face of these disturbances, propose a tool guaranteeing the proper functioning of the system in this context, and collect, annotate and increase the quantity of this new data for subsequent training?
Evidence of impact
Measuring impact using numerical data
> 98 % performance
– 50 % réduction in counting errors
Presentation of industrial impact
The Data Platform, developed as part of the Confiance.ai program, contributed to improving the robustness and operating reliability of Air Liquide’s models for forecasting demand over a month and automatic counting of objects under conditions adverse weather conditions. In particular, it made it possible to reduce the night counting error by half, to achieve precision performances above 98%:
- Thanks to data preprocessing consisting of eliminating snowflakes/transforming images from night to day which allowed the system to process, without additional training, this data as under normal learning conditions.
- Thanks to better control of this new data (study, visualization, characterization) and the completion of the training scenario base. Synthetic methods can make it possible to automatically annotate this data, or even generate more night/snow images that can be used by Air Liquide.
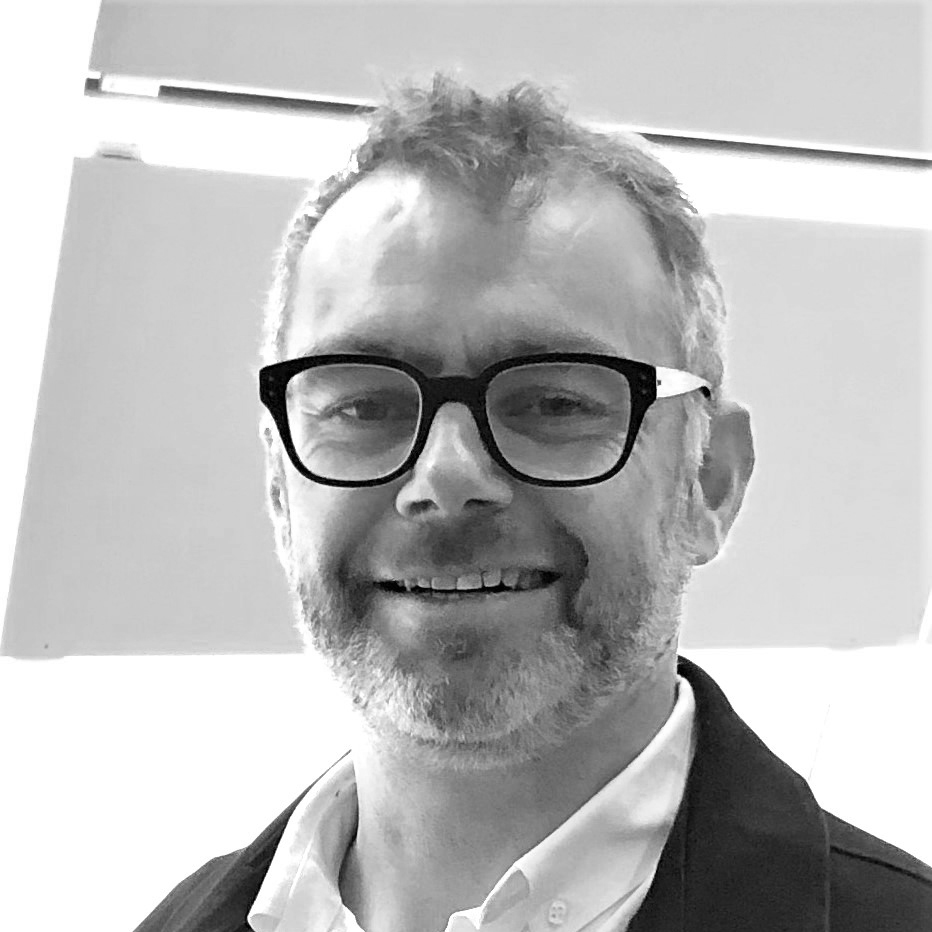
Quote representing CODIR
“Managing cylinders is of course at the core of Air Liquide operational excellence for packaged gas activities. Being able to correctly count the cylinders that come in and out from our filling centers is key to properly manage inventory and plan correctly daily or weekly operations. The developments made during Confiance.ai about confidence intervals enabled us to reach a sufficient level of trust and quality to propose a solution to our operations. This is now being tested in different parts of the world before full deployment.”
Fabien MANGEANT,
Scientific Director Computing & Data Sciences, Air Liquide